
- #Clarify image how to#
- #Clarify image full#
- #Clarify image registration#
- #Clarify image code#
- #Clarify image windows#
We noticed that the proposed approach achieved smooth organ boundaries. 3, all compared methods achieved reasonable deformation fields with the organ contours consistent with the fixed image.
#Clarify image registration#
Does it mean a CNN-based decoder was used to compute the registration field? 2, the Concat+Conv operations were required to compute DVF.
#Clarify image how to#

However, existing deep registration models, such as the diffeomorphic variant of the VM, were feasible to find the invertible registration fields. The authors claimed that the first two categories of methods failed to find the one-to-one correspondence between images. It is interesting to classify the existing registration methods as Fusion-first, Fusion-last, and cross-attention-based fusion.Please also refer to our Reviewer’s guide on what makes a good review: Please provide detailed and constructive comments for the authors.This paper has provided details about the models, datasets, and evaluation.
#Clarify image code#
Please be aware that authors are not required to meet all criteria on the checklist - for instance, providing code and data is a plus, but not a requirement for acceptance

Note, that authors have filled out a reproducibility checklist upon submission. Please comment on the reproducibility of the paper.Please rate the clarity and organization of this paper.
#Clarify image full#
There is a lack of architecture descriptions of the proposed full transformer-based registration model and the integration with existing registrations models. The discussions on the existing deep registration and transformer-based registration models are inappropriate. Please provide details, for instance, if you think a method is not novel, explain why and provide a reference to prior work.
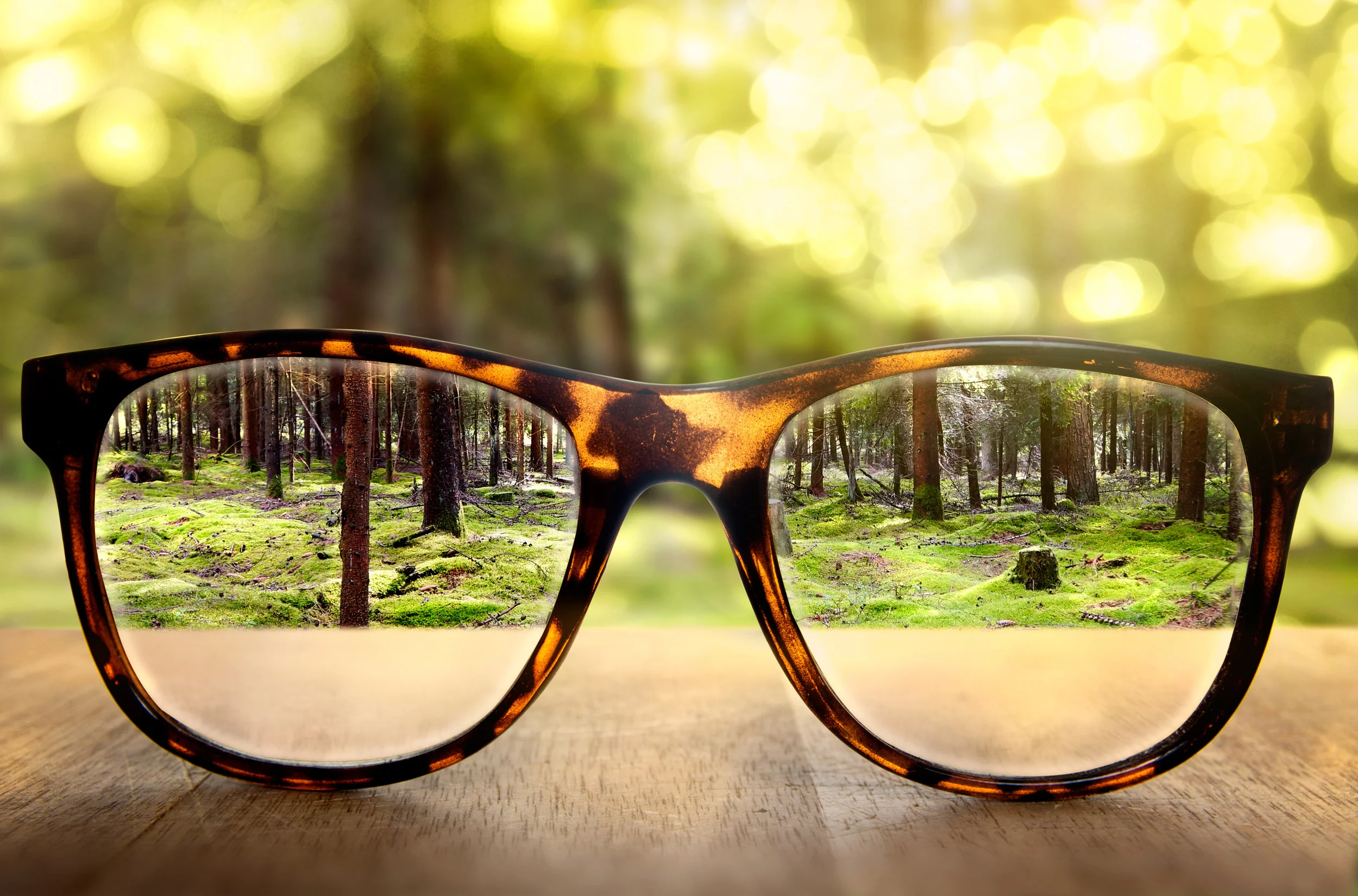
This work extended the Cross Attention Transformer (CAT) for communicationīetween a pair of features from moving and fixed images, promoting the features matching for image registration. Please provide details, for instance, if a method is novel, explain what aspect is novel and why this is interesting. Please list the main strengths of the paper you should write about a novel formulation, an original way to use data, demonstration of clinical feasibility, a novel application, a particularly strong evaluation, or anything else that is a strong aspect of this work.This paper proposed a full transformer architecture to extend the cross-attention transformer to establish the attention mechanism between images for the multi-level semantic correspondence. Please describe the contribution of the paper.We believe that our XMorpher has great application potential in more paired medical images. Without any bells and whistles, our XMorpher gives Voxelmorph 2.8% improvement on DSC, demonstrating its effective representation of the features from the paired images in DMIR.
#Clarify image windows#
3) It constrains the attention computation between base windows and searching windows with different sizes, and thus focuses on the local transformation of deformable registration and enhances the computing efficiency at the same time. 2) It advances the Cross Attention Transformer (CAT) blocks to establish the attention mechanism between images which is able to find the correspondence automatically and prompts the features to fuse efficiently in the network. 1) It proposes a novel full transformer architecture including dual parallel feature extraction networks which exchange information through cross attention, thus discovering multi-level semantic correspondence while extracting respective features gradually for final effective registration. Therefore, we advance a novel backbone network, XMorpher, for the effective corresponding feature representation in DMIR. However, the existing deep networks focus on single image situation and are limited in registration task which is performed on paired images. Jiacheng Shi, Yuting He, Youyong Kong, Jean-Louis Coatrieux, Huazhong Shu, Guanyu Yang, Shuo LiĪn effective backbone network is important to deep learning-based Deformable Medical Image Registration (DMIR), because it extracts and matches the features between two images to discover the mutual correspondence for fine registration. Paper Info Reviews Meta-Review Author Feedback Post-rebuttal Meta-Reviews Back to top
